A CADASTER report “Application of QSAR models for probabilistic risk assessment” has recently been accomplished. This report is a deliverable within the CADASTER project with an aim to show how to increase the use of non-testing information for regulatory decision whilst meeting the main challenge of quantifying and reducing uncertainty. The report contains examples of the integration of testing and non-testing information into assessment models for carrying out safety-, hazard- and risk assessments. The application of QSAR models for probabilistic risk assessment was discussed in respect to the characterization of uncertainty in QSAR predictions, the propagation of uncertainty in the assessment and sensitivity analysis of individual QSAR predictions with regard to their contributions in the overall risk assessment framework. Integration of QSARs in chemical safety assessment must acknowledge that treatment of uncertainty is context dependent, and uncertainty is to be interpreted in relation to the kind and quality of background information.
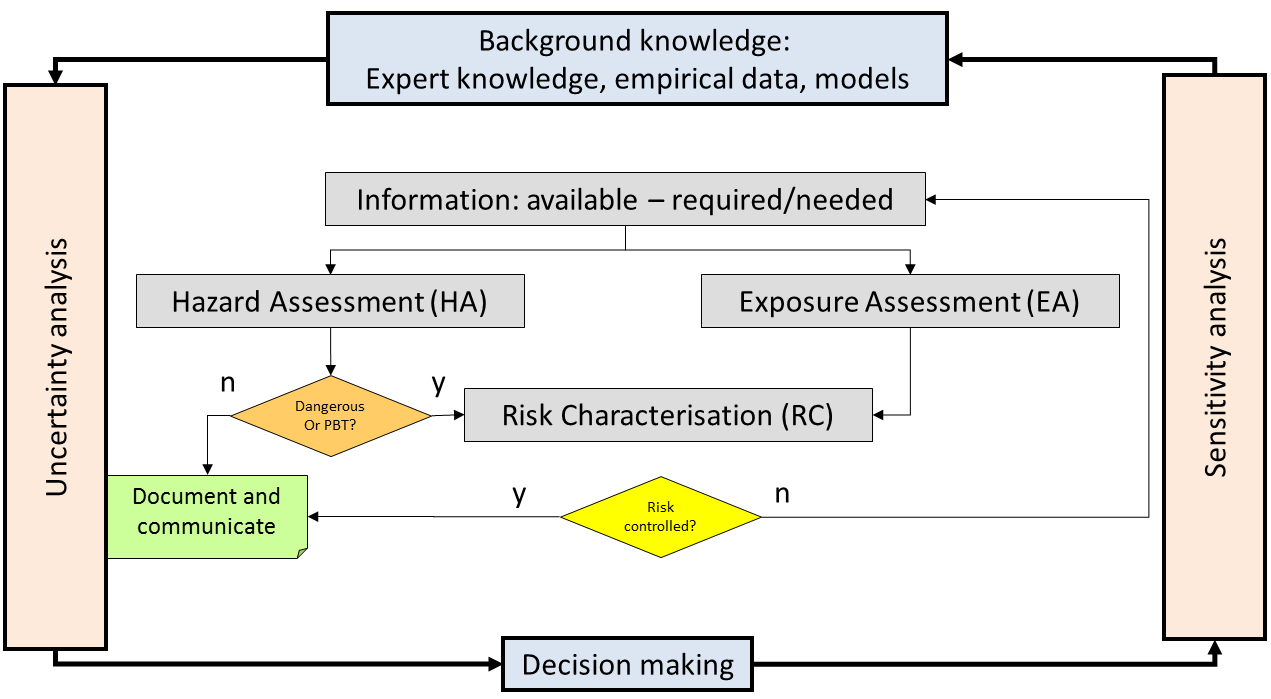
Two case-studies were used to demonstrate the computational framework for QSAR based risk assessment. The lesson learnt was that the application of QSARs in probabilistic risk assessment leads to questions such as:
- Are there any QSAR data available to use as weight-of-evidence of a chemico-specific input parameter to the exposure and effect assessments?;
- Which validated algorithm for prediction and approach for predictive inference will be used to support the uncertainty analysis?
- Is a QSAR prediction reliable enough to support the intended decision making?
The first case-study was an uncertainty and sensitivity analysis of QSAR and QSPR based-risk assessment on five Triazoles. Soil sorption partition coefficients, solubility, melting point, vapor pressure and hydroxyl radical reaction in air were predicted with identified QSPRs, and no effect concentrations with available QSARs developed for Triazoles. Parameter uncertainty was treated as a probability distribution for the chosen QSAR algorithm, for example a t-distribution when the QSAR algorithm was an ordinary least square regression. Exposure and effect assessment were carried out in the multimedia fate model Simplebox, and uncertainty was propagated by Monte Carlo simulation. Uncertainty analysis showed that the maximum permissible emissions to agricultural soil were highest for the Triazoles Bromuconazole and Difenoconazole. Sensitivity analysis, aimed to find sources of uncertainty with a large influence on the uncertainty in assessment output, found that uncertainty in maximum permissible emissions to agricultural soil was mainly determined by uncertainty in the soil sorption partition coefficient, the biodegradation in water and the toxicity.
The second case-study compared the discrepancy between non-testing versus testing-based risk assessments on three polybrominated diphenyl ethers. Exposure (PEC) and effect (PNEC) were assessed based on QSPR and QSAR predictions, treated in the same way is the first case-study except that variability in effect was considered through a species sensitivity distribution. Whenever available, parameters for the exposure assessment and species effect concentrations were replaced by information from experimentally tests on the chemicals in question. Discrepancies between non-testing and testing-based risk assessments were evaluated by studying the range of emissions for which the resulting decisions were different. We found that non-testing versus testing based risk assessments were different, but this difference depend to a large extent to how uncertainty is dealt with. The difference is small when predictions are precise, i.e. providing a good but balanced coverage of the experimental values, non-testing information are useful complement to reduce uncertainty in existing testing information of effects. However, there is uncertainty in experimental data which are not accounted for in QSAR modeling. The larges discrepancies could however be related to the use of uncertainty factors. For example, PNEC values were given a different treatment of uncertainty depending on the number of species with available information, where QSAR predictions increased the number of covered species. Also, experimental information was available for acute effects, whereas QSAR predictions were available for chronic effects, was another cause for difference in the treatment of uncertainty.
With respect to treatment uncertainty in QSAR predictions for probabilistic risk assessment the conclusions in the report are that:
- The integration of QSARs into probabilistic risk assessment is possible given proper assessments of predictive uncertainty and predictive reliability. Predictive uncertainty and reliability are identified to inform the characterization of parameter and model uncertainty, two kinds of uncertainty to be identified in probabilistic risk assessment. Uncertainty in probabilistic chemical safety assessment can roughly be divided in to three categories: parameter uncertainty, model uncertainty and scenario uncertainty. According to ECHA scenario uncertainty is “the uncertainty in specifying the scenario(s) which is consistent with the identified use(s) of the substance”. Scenario uncertainty is of less relevance for the uncertainty related to a QSAR. The other two terms, parameter and model uncertainty, are not frequently used in QSAR modeling. Instead a distinction lies between predictive uncertainty and predictive reliability.
- Probabilistic risk assessment is supported by QSAR predictions derived from Bayesian predictive inference. Predicting must be done with care, and the use of different bases for predictive inference is possible when a QSAR is treated as a scientific based hypothesis supported by empirical data. In other words, the suggestion is to treat QSAR data that has been validated for its predictive performance as the QSAR to base predictions on. It is up to the assessor to choose an appropriate method for predictive inference, given that performance measures of predictivity do not deviate to much from the measures in the peer-reviewed validation. This will result in a more flexible use of QSARs with a possibility of updating as long as new QSAR data becomes available.
- The extent of extrapolation in a QSAR prediction influences predictive error and predictive reliability, and the domain of applicability is from an applied perspective context dependent and considered in the treatment of uncertainty. A separation between predictive uncertainty and predictive reliability makes it possible to both apply models and discuss their reliability in a constructive way.
This report focuses on predictions of chemical specific properties and activities to replace testing information in chemical regulation. Statistical inference may have different purposes, and when QSARs are applied to support decisions making based on unobserved quantities such as in risk assessment, drug development, or experimental design, the statistical problem is to make predictions, referred to as predictive inference. Predictive science involves the use of a belief system about observables in science, and a philosophy of scientific methodology that implements that belief system. Predicting should be done with care and there are (solvable) practical problems when the purpose of statistical inference changes from inference on models to inference on predictions.
The report discusses three kinds of philosophies for predictive inference of relevance for the application of QSARs in probabilistic risk assessment. Sampling Theory estimate predictive uncertainty based on a representative sample. Such (frequentist) inference rests upon assumptions of independent and, for example, identically distributed observations, in combination with a probabilistic assumption of uncertainty. Under violence of any of these assumptions, appliers of frequentist inference run into problems.
The Bayesian paradigm for inference
assign, instead of
assume, a probabilistic model for observations, and assign models for uncertainty in parameters (so called priors). Bayesian inference uses Bayes rule to update expert knowledge with information in empirical observations. The result is a well-defined probabilistic model of uncertainty. In cases of doubts, the caveat is the necessity to choose priors and probabilistic models (likelihoods). For example, there is no need to check an assumption of normality of errors (as in the frequentist case), as this is assigned through expert judgment.
The third alternative is to assign a probability distribution for predictive uncertainty based on expert judgment only. This can for example, be based on experience of experimental testing, or based on combinations of different sources of information.
Sampling Theory and solid expert judgment can be seen as extremes kinds of Bayesian inference; the first as Bayesian inference with non-informative priors (expressed simplistically, but it is more difficult than that); the second as Bayesian inferences with only priors. Therefore the recommendation we give is to use Bayesian inference as the statistical philosophy for predictive inference when QSARs are applied in probabilistic risk assessment.